In deterministic quantum computing with one qubit (DQC1), a non-universal quantum computing model used in supervised machine learning, a recent study has examined the possible advantages of quantum coherence and quantum discord.
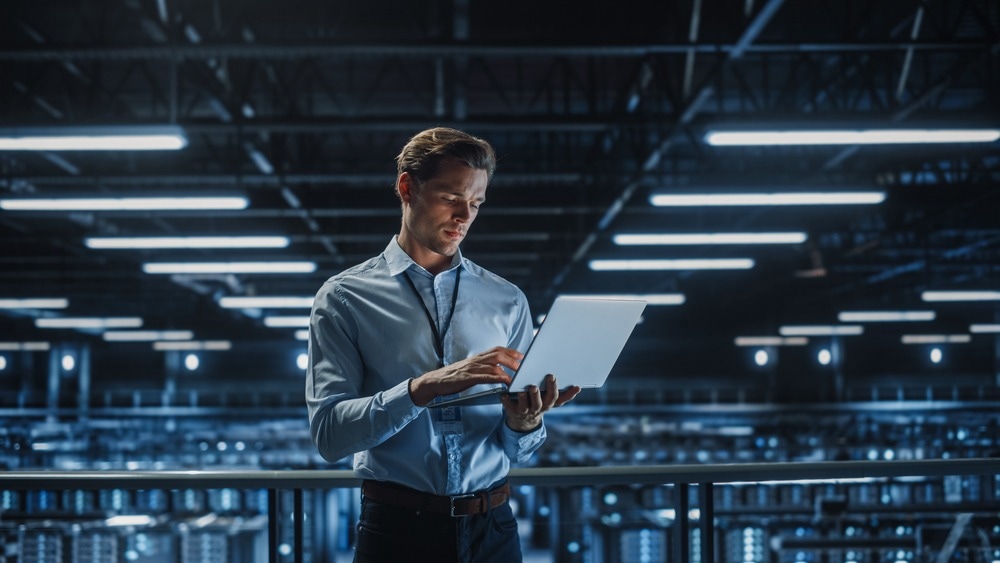
Image Credit: Gorodenkoff/Shutterstock.com
Developments in the management and reduction of noise and decoherence in recent times have made it possible to create intermediate-scale quantum systems made up of hundreds of qubits.
Due to their capacity to sustain quantum entanglement, these machines are currently not fault-tolerant, although there is strong evidence that they have higher computational capabilities when compared to classical supercomputers. It is anticipated that quantum hardware will become increasingly important in a number of domains, including quantum machine learning (QML), quantum chemistry, and quantum simulations.
Machine Learning with DQC1
Recent research investigates the effective estimation of complex kernel functions by supervised machine learning using the DQC1 model. The study is conducted on IBM hardware and looks at hardware noise, quantum discord, and coherence consumption.
The DQC1 model has been used in a variety of physical settings since it was first presented in the context of nuclear magnetic resonance (NMR) quantum information processing.
A lot of research has been done on the DQC1 model to look into possible applications of quantum resources other than entanglement in quantum computing.
Quantum Coherence and Quantum Discord
The capacity of quantum systems to preserve a steady phase relationship between various quantum states is known as quantum coherence. To fully realize the potential of quantum computing, quantum coherence must be preserved.
Quantum discord is a measure of nonclassical connections between two subsystems of a quantum system in quantum information theory. The discrepancy between the total quantum mutual information and the classical mutual information of the subsystems is known as quantum discord, which is a generalization of the classical idea of mutual information.
Analyzing quantum discord and coherence, the study attempts to show that the creation of discord is made possible by the consumption of quantum coherence.
The research effort looked at the relationship between the formation of discord in DQC1 and coherence consumption and found that quantum discord is limited by the quantum coherence consumed in the control qubit.
The relationship is confirmed by the IBM hardware results.
Support Vector Machines and Kernel Machines
Support vector machines (SVMs), also known as support vector networks, are supervised learning models in machine learning that analyze data for regression and classification along with corresponding learning algorithms.
The most well-known member of the class of pattern analysis algorithms in machine learning, known as kernel machines, is the support-vector machine (SVM). These techniques entail solving nonlinear problems with linear classifiers.
By considering the feature map as a quantum state that may be created by a quantum circuit and the kernel function as the inner product between the corresponding quantum states, the fundamental concepts of SVM can be expanded to the quantum realm.
DQC1 can be related to the kernel approach because of the flexibility in selecting the unitary operator.
Testing on IBM’s Quantum Processor
IBM's Qiskit open-source software interface was used to test the approach on the quantum processor.
IBM's ibm_perth qubit superconducting quantum processor was used for the demonstration. It is made up of transmon qubits with set frequencies that are coupled based on the coupling map.
The three stages of implementation are separated. Using the quantum state tomography package in Qiskit and repeating each measurement 8000 times, first the test was run for all pairs of training data in order to obtain the corresponding density matrix for the control qubit and, consequently, the corresponding kernel function. After obtaining the kernel function on the quantum hardware, the ideal separating hyperplane was determined by using the classical SVM.
Results and Outlook
Based on the average purity of the encoded states, the fidelity quantum kernel model's generalization error upper bound was obtained. As the same work has shown, when moving data into quantum states, a noisier encoding procedure might result in a lower training performance.
The findings are consistent with the hypothesis that raising the control qubit's purity improves the DQC1 kernel model's accuracy. The results show that the DQC1 kernel architecture that has been developed offers a solid theoretical foundation for investigating the relationship between generalization error and coherence consumption.
Another proposal looked into using the constrained computational model DQC1 for supervised machine learning tasks. The DQC1 model does not use quantum entanglement in the computation; instead, it depends on mixed states, in contrast to the normal universal computational model. A test of the DQC1 model's aptitude for handling supervised machine learning issues for a few traditionally challenging kernels has been provided. Since one needs to test only the control qubit, the protocol required more gates than other similar protocols, yet it still produced a relatively good classification accuracy.
This study provides a proposal that emphasizes the advantages of quantum discord over entanglement when noise is present. It also demonstrates the computing capability of a single-qubit as a universal classifier in a larger context.
More from AZoQuantum: Why We Need Generalized Quantum Standards
References and Further Reading
Karimi, M., Javadi-Abhari, A., Simon, C. et al. The power of one clean qubit in supervised machine learning. Sci Rep 13, 19975 (2023). https://doi.org/10.1038/s41598-023-46497-y
Havlíček, V. et al. Supervised learning with quantum-enhanced feature spaces. Nature 567, 209–212 (2019).
Huang, H.-Y. et al. Quantum advantage in learning from experiments. Science 376, 1182–1186 (2022).
Preskill, J. Quantum computing in the Nisq era and beyond. Quantum 2, 79 (2018).
Disclaimer: The views expressed here are those of the author expressed in their private capacity and do not necessarily represent the views of AZoM.com Limited T/A AZoNetwork the owner and operator of this website. This disclaimer forms part of the Terms and conditions of use of this website.