Researchers from the clinical stage AI-driven drug discovery company Insilico Medicine (Insilico) show how quantum computing can be incorporated into the study of living organisms to provide more light on biological processes like aging and disease in a new study published in WIREs Computational Molecular Science.
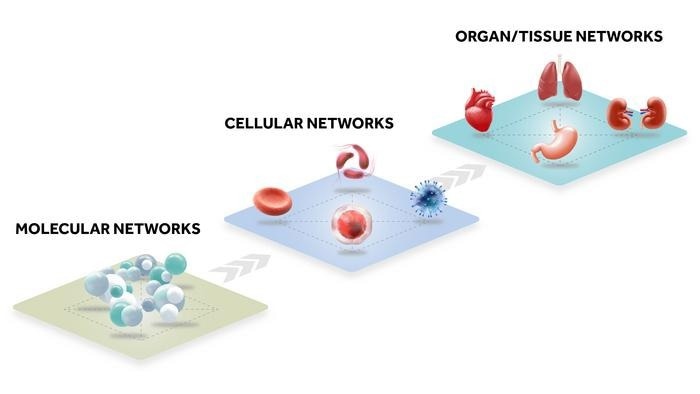
Biological networks are interconnected. Just as knowing the ingredients alone is not sufficient to understand how to prepare a dish, understanding only the list of genes or proteins is insufficient to comprehend how they interact. Image Credit: Insilico Medicine
Research by Insilico, Foxconn Research Institute, and the Acceleration Consortium at the University of Toronto effectively illustrated the potential benefits of quantum generative adversarial networks in generative chemistry and was published in May 2023. The American Chemical Society’s Journal of Chemical Information and Modeling reported their findings.
Insilico researchers have published a new study that details the current developments in physics-guided AI and paints a comprehensive picture of how merging techniques from AI, quantum computing, and the physics of complex systems might help researchers create new understandings of human health.
They write that although AI has proven to be a valuable tool in assisting researchers in processing and analyzing vast, intricate biological datasets to identify novel disease pathways and establish cellular connections between aging and disease, it still encounters difficulties when attempting to apply these discoveries to more intricate interactions within the body.
The researchers highlight that multimodal modeling techniques that can handle three major areas of complexity—the complexity of scale, the complexity of algorithms, and the growing complexity of datasets—are essential for scientists to gain a complete understanding of the inner workings of living organisms.
While we are not a quantum company, it is important to utilize capabilities to take advantage of the speed provided by the new hybrid computing solutions and hyperscalers. As this computing goes mainstream, it may be possible to perform very complex biological simulations and discover personalized interventions with desired properties for a broad range of diseases and age-associated processes. We are very happy to see our research center in the UAE producing valuable insights in this area.
Alex Zhavoronkov, Ph.D., Study Co-Author, Founder and Co-CEO, Insilico Medicine
Living systems have biological processes that span from cells to organs to the entire body, involving numerous intricate intersystem relationships. Working at several scales at once is necessary for the interpretation of these processes. Furthermore, biological data is now accessible at previously unthinkable levels.
Just two examples of human genetic variation catalogs exist: the UK Biobank, which has entire sequences from 500,000 genomes of British volunteers, and the 1000 Genomes Project, which has found over 9 million single nucleotide variants (SNVs). Massive processing and analysis power is required.
The researchers claim that quantum computing is in a unique position to complement AI techniques as it enables simultaneous interpretation at several layers of the biological system. Qubits are far more capable and faster computers than classical bits since they can store both 0 and 1 concurrently, while classical bits can only store 0 or 1.
The authors point out that significant progress in quantum computing has already been made, such as IBM’s recent introduction of a utility-scale quantum processor and its first modular quantum computer, which is already in use.
Finally, the authors advocate for a physics-guided AI strategy to better understand human biology, highlighting an emerging field that blends physics-based and neural network models as currently underway.
Scientists can get a deeper understanding by merging technologies from AI, quantum computing, and complex system physics.
The study authors concluded, “the collective interactions of smaller-scale elements within a cell, organism, or society generate emergent characteristics that can be observed at larger scales and levels of reality.”
Journal Reference
Kao, P.-Y., et. al. (2024) Exploring the Advantages of Quantum Generative Adversarial Networks in Generative Chemistry. Journal of Chemical Information and Modeling. doi:10.1021/acs.jcim.3c00562