The efficacy of artificial intelligence heavily hinges on processing extensive datasets, frequently involving the execution of complex mathematical operations. Quantum computing stands poised to substantially expedite these processes, offering numerous advantages to the realm of AI. Quantum computers can play a pivotal role in advancing AI algorithms, pushing the boundaries of problem-solving to the extent of our creative thinking and our capacity to articulate the problem at hand.
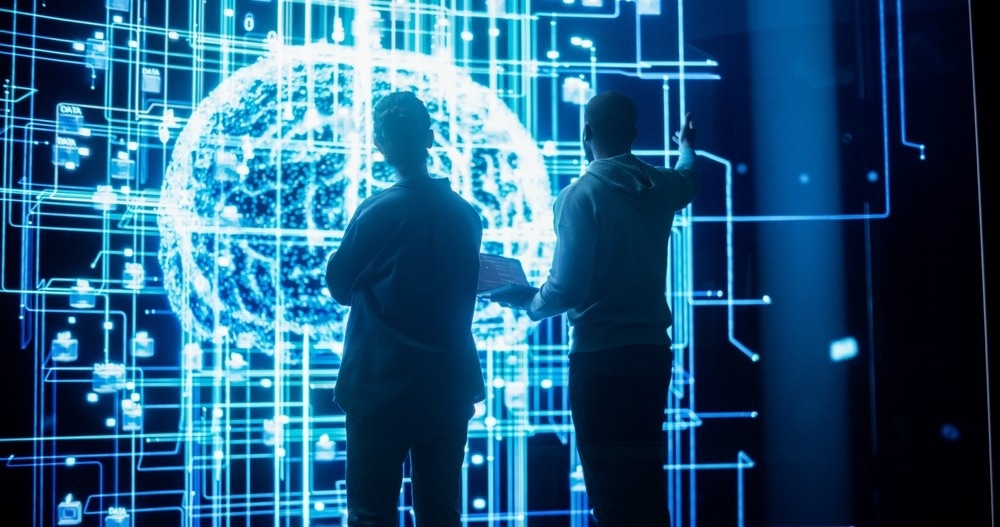
Image Credit: Gorodenkoff/Shutterstock.com
An Interconnection of Quantum Computing and AI
Contemporary deep learning and AI algorithms find application in diverse commercial projects, including self-driving cars and image-processing software applications. The effective implementation of these algorithms necessitates extensive training using substantial datasets. Quantum computing holds the promise to substantially expedite the training process of neural networks, a pivotal facet of deep-learning and AI algorithms.
Quantum computers utilize quantum parallelism to process extensive datasets simultaneously, leading to quicker convergence and the creation of more precise models. This strategy involves deconstructing AI and deep-learning challenges in areas where entanglement is minimal, executing operations on multiple quantum processors, and subsequently amalgamating the outcomes through classical means.
The potential of quantum computing to improve natural language processing (NLP) techniques lies in its ability to attain a more profound comprehension of context, sentiment, and nuance within textual data.
This progress stands to enhance the capabilities of AI-driven applications such as chatbots, translation tools, and other language-related applications. Furthermore, the accelerated data processing by quantum processors has bolstered the efficacy of computer vision algorithms, fostering advancements in image recognition, object detection, and facial recognition.
Applications of Quantum-Powered AI Algorithms
The future of data processing is often associated with quantum computing technology, prompting significant investments from major companies such as IBM, Google, and Microsoft. The considerable speed improvements and enhanced power efficiency provided by quantum computers have reimagined the potential of artificial intelligence and machine learning, fostering revolutionary progress across diverse industries in the contemporary landscape.
The financial markets extensively used quantum processors and computing processes for running AI and ML algorithms. Various financial prediction AI models are run using quantum computers and make forecasts about financial markets.
Advancements in deep learning are anticipated to contribute to a better understanding of quantum mechanics. Simultaneously, fully developed quantum computers have the potential to outperform traditional computers, especially in tasks related to data pattern recognition. For instance, IBM's quantum research team has observed that entangling qubits in a quantum computer during a data-classification experiment led to a significant reduction in the error rate compared to un-entangled qubits. This highlights the potential synergy between quantum computing and machine learning techniques.
Quantum machine learning (QML) applications span across quantum simulation and quantum optimization, often involving hybrid classical-quantum algorithms that leverage classical neural networks. In molecular simulation, QML is employed for drug discovery, material development, and climate modeling. The energy sector utilizes QML for tasks such as energy grid optimization, energy demand prediction, energy storage, distribution, and the integration of renewable energy sources. These applications showcase the potential of quantum computing in addressing complex problems in various domains.
What are Some Useful Quantum Computing Techniques Used in AI Fields?
Among the various fields in which quantum computing is playing a pivotal role in the expansion of AI, researchers have mentioned a few techniques in an article published at the 2020 International Conference on Information Networking (ICOIN).
The Quadratic Unconstrained Binary Optimization (QUBO) model proves to be a valuable framework for expressing combinatorial optimization problems (COPs). These problems, characterized by discrete decision variables and a finite search space, are prevalent in various fields. Quantum annealing, based on the Ising model in physics, has found relevance in solving QUBO problems. Additionally, the study of neuromorphic computing further expands the scope of applications for the QUBO model, indicating the diverse and impactful intersections between quantum computing and computational paradigms like AI.
Quantum Computers Enabling AI-Based Mobile Network Improvements
The availability of cloud-based quantum computing platforms has ignited a new wave of research and development, fostering innovative solutions across diverse domains. In the realm of mobile networks, which are becoming progressively intricate, the demand for powerful optimization methods and computing platforms is growing. The optimization of parameters in mobile networks involves highly complex computational problems, and the performance of these networks relies on the computational intelligence and capabilities of the automation platform. Quantum computing, with its potential for handling complex problems, is emerging as a promising avenue for addressing challenges in optimizing mobile networks.
Indeed, the integration of artificial intelligence (AI) with quantum computing holds great promise, particularly in the context of 5G and beyond. Researchers are exploring the application of quantum computing to address combinatorial optimization problems, particularly constraint satisfaction problems (CSPs). The discussion includes the formulation of radio access network (RAN) optimization use cases as CSPs, which can be translated into quadratic unconstrained binary optimization (QUBO) problems.
In a specific application, researchers formulated the Remote Radio Head (RRH) Signal Interference (RSI) assignment as a QUBO problem in an article published in the 2021 IEEE 32nd Annual International Symposium on Personal, Indoor, and Mobile Radio Communications (PIMRC), leveraging data from a commercial mobile network.
They utilized a cloud-based quantum computing platform for solving the problem, demonstrating that quantum annealing solvers can successfully assign conflict-free RSIs. It's noted that the results can vary based on the type of quantum computer used, but the proposed framework exhibits flexibility and significant potential for leveraging quantum computing in the automation of mobile networks.
Classical algorithms, especially those used for vector problems in high-dimensional spaces, often encounter challenges in terms of computational efficiency. Quantum algorithms, drawing from principles in quantum mechanics, have the potential to offer significant speed-ups in solving such problems.
The recent advancements in Open Quantum Systems, involving the study of quantum systems interacting with external environments, show promise in improving the robustness and decision-making capabilities of AI algorithms. The convergence of quantum computing and AI holds the potential for widespread technological innovation across various industries and problem domains.
More from AZoQuantum: How the UK is Steering International Quantum Standards
References and Further Reading
Elarns, (2023). How is Quantum Computing Used in AI?. [Online]
Available at: https://medium.com/@tali79843/how-is-quantum-computing-used-in-ai-e84458d13f7a
Gossett, S., (2022). 10 Quantum Computing Applications and Examples. [Online]
Available at: https://builtin.com/hardware/quantum-computing-applications
Hyscaler, (2023). How Quantum Computing is transforming AI: The Quantum Leap. [Online]
Available at: https://hyscaler.com/insights/how-quantum-computing-transforms-ai/
Parente, D., (2023). The Applications of Quantum Computing in Deep Learning and Artificial Intelligence. [Online]
Available at: https://medium.com/daniel-parente/the-applications-of-quantum-computing-in-deep-learning-and-artificial-intelligence-2d4d24b3312a
QuEra Computing Inc., (2023). Top Applications Of Quantum Computing for Machine Learning. [Online]
Available at: https://www.quera.com/blog-posts/applications-of-quantum-computing-for-machine-learning
Walia, M., (2022). Is Quantum Computing the Future of Artificial Intelligence?. [Online]
Available at: https://www.analyticsvidhya.com/blog/2022/04/is-quantum-computing-the-future-of-artificial-intelligence/
Jaeho, C. et. al. (2021). "The Useful Quantum Computing Techniques for Artificial Intelligence Engineers," 2020 International Conference on Information Networking (ICOIN) pp. 1-3. Available at: https://doi.org/10.1109/ICOIN48656.2020.9016555
Furqan, A. et. al. (2021). Quantum Computing for Artificial Intelligence Based Mobile Network Optimization, 2021 IEEE 32nd Annual International Symposium on Personal, Indoor and Mobile Radio Communications (PIMRC). pp. 1128-1133. Available at: https://doi.org/10.1109/PIMRC50174.2021.956933
Disclaimer: The views expressed here are those of the author expressed in their private capacity and do not necessarily represent the views of AZoM.com Limited T/A AZoNetwork the owner and operator of this website. This disclaimer forms part of the Terms and conditions of use of this website.