Quantum technology and artificial intelligence (AI) are among the most revolutionary disciplines that have attracted considerable interest in recent years. Quantum physics has altered our understanding of the natural world, and one of its subdivisions, quantum mechanics, has produced precise and uniform theoretical findings.
Quantum computing (QC) is the application of quantum mechanics to the calculation process. AI deals with training machines and computers to perform repetitive tasks and learn novel methodologies based on test data available. The relationship between AI and quantum technology has led to modern innovative systems involving a mix of both algorithms providing sustainable solutions in various fields.
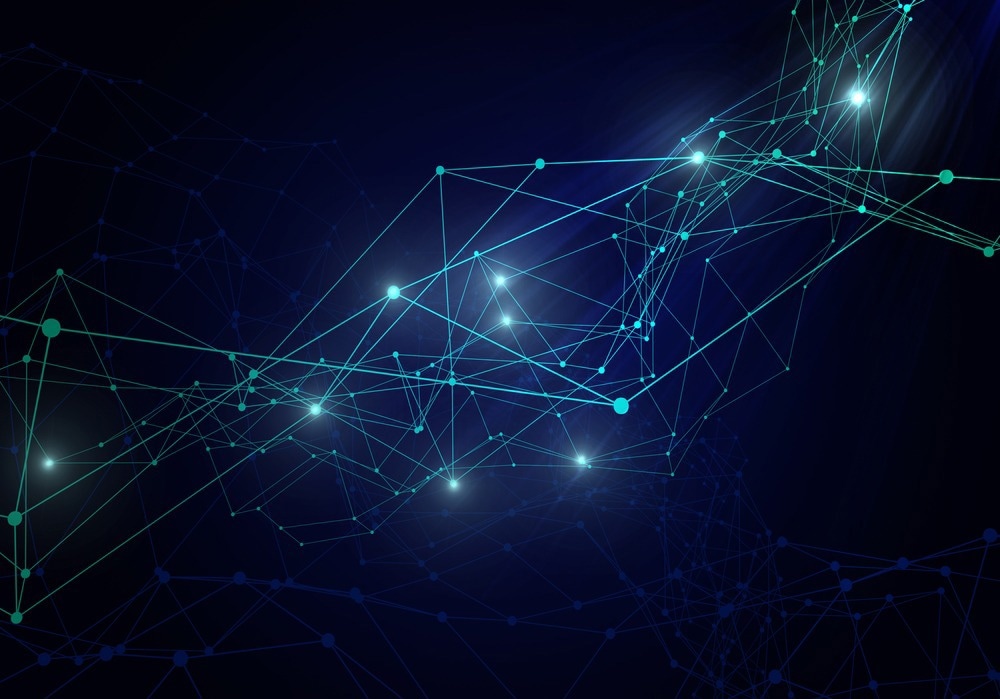
Image Credit: Max100ka/Shutterstock.com
Quantum Machine Learning
The growing diversity of data sets and the requirement to extract data from them have led to the widespread application of Machine Learning (ML) in numerous fields. Quantum Control and Machine Learning (QCML) have converged to form a new discipline, Quantum Machine Learning (QML), which combines principles from both disciplines to produce augmented solutions, either by enhancing ML algorithms or quantum investigations, or both.
As per an article published in Neurocomputing, Quantum auto-encoders, analogous to auto-encoders of conventional Machine Learning, have been explored in the past few years as a method to efficiently utilize the resources in quantum computation. The use of traditional ML algorithms in the fields of quantitative experiments and quantum data is currently a hot topic in the scientific community.
One of the earliest promising outcomes involved the incorporation of Reinforcement Learning (RL) into adaptable quantum measurement, where RL-based control of quantum operations outperformed conventional greedy techniques. RL has also been utilized in the area of quality control for continuous nonconvex minimization in circuit simulations and ultra-cold-atom experiments.
The most difficult aspect of recovering quantum details involves devising an approach to minimize measurement costs while obtaining the relevant data. Since AL takes into consideration the cost of categorization, i.e. the loss of fidelity induced by evaluation, it is compatible with the theoretical framework of quantum measurement, which seeks to minimize computations.
Another relatively prevalent application of RL to QC is measure control. The significance of this problem derives from the fact that it may be challenging to obtain data embedded in a quantum state for any type of processing. Due to Active Learning's (AL) appealing qualities in these circumstances, there have been recent efforts to implement it for quantum testing within this framework.
Application of Quantum ML in the Biomedical Domain
Quantum machine learning has grown into a prevalent and efficient method for analyzing data and data segmentation in a variety of domains. Quantum ML has found numerous applications, especially in the biomedical field, as per the latest article in IEEE Access. The renowned scientific discipline in which QML plays a vital role, Omics, is expanding rapidly. The term ''omics'' signifies data gathered through genetics.
Chromosomal sequence, binding protein forecasting, and genomic data are QML techniques that are utilized to anticipate symptoms from the genome.
A novel swarm intelligence algorithm for modal and multi-classification of Omics (Colon and Brain tumor) databases (binary and multi-classification) has been designed. Classifying the tiny gene subsets with the Quantum moth fame optimization algorithm (QMFOA).
The quantum-inspired Helstrom Quantum algorithm has been used in the biomedical imaging context of clonogenic test evaluation for the segmentation of cell colonies. During the worldwide epidemic, QML algorithms were applied to COVID-19 X-rays of the lungs to categorize the disease.
What are Bayesian Quantum Neural Networks?
The latest article in IEEE provides information about Bayesian quantum neural networks. The classification algorithm Nave Bayes is used for massive amounts of data. Recent research focuses on the effects of Bayesian Estimation on QNNs, which are broken down into two key elements of mathematical learning: model capacity and adaptability, and predictive accuracy.
The Bayesian QNN's attractive feature is marginalization, which improves accuracy and calibration.
The researchers utilized two variational inferences to teach Bayesian quantum ML models to classical Bayesian NN. In addition to achieving results comparable to the frequentist approach, the suggested methods also captured the ambiguity in the model's inferences.
Hybrid Classical Quantum Deep Learning in the Semiconductor Industry
With the accelerated advancement of AI and technology for autonomous driving, it is anticipated that microchip consumption will increase substantially. Deep learning as a trending computer vision technology has been implemented in a variety of fields.
The researchers presented an article in the Proceedings of the IEEE/CVF Conference on Computer Vision and Pattern Recognition in which they suggested hybrid classical-quantum deep learning (HCQDL) founded on quantum circuits for various forms of imperfection examination. The quantum computing layer is implemented through different quantum circuits constructed in a continuous-variable architecture, encoding quantum data with continuous degrees of freedom.
The classical level, quantum layer, and entire connection layer make up HCQDL. The self-proliferation and self-attention (SP&A) block is used to efficiently derive feature maps while implementing the classical layer. The quantum layer consists of the quantum circuitry that may produce extremely complicated elements whose traditionally intractable calculations are generated.
The quantum layer is carried out by numerous quantum circuits based on the architecture of continuous-variable.
The work emphasizes the innovations of quantum deep learning, and the model demonstrated the generalizability of convolutional layers within a typical CNN architecture.
The experimental outcomes demonstrated that the framework surpasses extant deep learning methods. Moreover, based on recent advancements, it is anticipated that quantum advantage will be attainable within the next 5 to 10 years.
Other Major Interactions
Quantum cryptography offers an appealing approach to enhancing the security of AI systems, protecting classified information, and preventing illicit access and modification. Through the use of quantum key distribution, AI systems can utilize powerful encryption algorithms and methods of communication, ensuring the safety of sensitive data. Notably, this strategy is a priceless protection against malicious assaults on AI data. The innate susceptibility of AI systems can be substantially reduced by employing encryption techniques that are immune to quantum computing-based attacks. Moreover, the incorporation of quantum sensing techniques provides a prospective route toward improving gathering data in AI applications.
Challenges
AI scientists and programmers are expecting quantum cloud platforms to contribute an essential part in expanding the utilization of quantum computing resources. Owing to the intrinsic fragility of qubits, correcting errors is a fundamental obstacle in quantum computing. To circumvent hardware difficulties and enhance the dependability of quantum computing, it is necessary to create resilient error correction codes and fault-tolerant designs.
To summarize, the integration of these novel technologies is providing sustainable solutions in the modern Industry 4.0 era. For instant commercialization and research, technical and hardware limitations, ethical concerns, and knowledge gaps must be addressed.
More from AZoQuantum: Google Quantum AI Presents New Superconducting Quantum Processor
References and Further Reading
Martín-Guerrero, D., & Lamata, L. (2022). Quantum machine learning: A tutorial. Neurocomputing, 470, 457-461. Available at: https://doi.org/10.1016/j.neucom.2021.02.102
Maheshwari, D. et. al. (2022). Quantum machine learning applications in the biomedical domain: A systematic review. IEEE Access. Available at: https://doi.org/10.1109/ACCESS.2022.3195044
Nguyen, N., Kwang C. (2022). "Bayesian quantum neural networks." IEEE Access 10 (2022): 54110-54122. Available at: https://www.doi.org/10.1109/ACCESS.2022.3168675
Yang, F., & Sun, M. (2022). Semiconductor defect detection by hybrid classical-quantum deep learning. In Proceedings of the IEEE/CVF Conference on Computer Vision and Pattern Recognition. (2323-2332). Available at: http://openaccess.thecvf.com/content/CVPR2022/html/Yang_Semiconductor_Defect_Detection_by_Hybrid_Classical-Quantum_Deep_Learning_CVPR_2022_paper.htm
Disclaimer: The views expressed here are those of the author expressed in their private capacity and do not necessarily represent the views of AZoM.com Limited T/A AZoNetwork the owner and operator of this website. This disclaimer forms part of the Terms and conditions of use of this website.